Current Projects
Securing High-Density Urban Airspaces
This project will establish a security framework and algorithmic foundations to safeguard high-density urban airspace against cyberattacks. We will focus on attacks on heterogeneous airspace operations, which include both electric vertical take-off and landing (eVTOL) aircraft and small unmanned aerial systems (sUAS). The outcome of the project will be security-aware improvements on the current advanced air mobility (AAM) and UAS traffic management (UTM) system architecture, avionics software, ground control station (GCS) software, and aircraft operations.
Safety, Certification and Trust of Machine Learning and Artificial Intelligence
We are working on the certification, auditing and regulation of machine learning and AI systems in safety critical applications such as aviation, transportation and robotics. Our focus is on (1) how to certify a data-driven learning-based system? (2) how to certify a perception, prediction and/or decision making system with neural net(s)? (3) how to build trust for these ML/AI systems when human in or on the loop?
Autonomy in Urban Air Mobility (UAM) and UAS Traffic Management (UTM)
This research focuses on both vehicle autonomy and airspace autonomy to achieve operation safety, efficiency, and scalability for urban air mobility (UAM) and UAS traffic management (UTM), which includes arrival management, computational guidance, separation assurance, conflict resolution, autonomous air traffic control, strategic flow management and fleet dispatch.
Vertiport Automation and Decision Support
We have been working with our collaborators and partners to develop a vertiport management system to support real vertiport operations in New York City. We have built the digital twin of the NYC advanced air mobility (AAM) network.
Stochastic and Robust Optimization for Multiple Constrained Resources Air Traffic Flow Management
Our team is building stochastic mathematical programming models for sequential decision making under uncertainty in the newest Federal Aviation Administration (FAA) air traffic flow management tools.
Deep Reinforcement Learning (DRL) and Multi-Agent Reinforcement Learning (MARL)
We are creating models and algorithms to beat the state-of-the-art performance in deep reinforcement learning (DRL) and multi-agent reinforcement learning (MARL).
Past Projects
Towards an Intelligent Low-Altitude UAS Traffic Management System (NSF)
The research provided theoretical foundations to support UAS pre-departure traffic coordination and en route traffic management in low-altitude airspace.
Urban Air Mobility (A^3 by Airbus)
In order to enable on demand air transportation in an urban setting, our research group was working with Airbus A^3 teams on new concept of operations, modeling and simulation, and algorithm design and analysis to provide safe, efficient, sustainable and intelligent solutions for urban on-demand air transportation.
Passenger Direct Share Forecast (FAA)
The objective of this FAA project was to build machine learning based predictive models to forecast the direct passenger percentages for all the origin-destination airport pairs in the United States. The newly developed model is expected to replace the current FAA forecasting model.
Aviation Weather Impact on Flight Operations (Collins Aerospace)
Our group was working with Rockwell Collins Advanced Technology Center to study the flight en route time variation under different convective weather events. Machine learning algorithms are being developed and analyzed by mining the large-scale nationwide flight data sets (AOTP) and meteorology data sets (ASOS, HRRR, NEXRAD). The resulted predictive model is expected to provide estimation for aircraft en route time before departure given weather forecast, which is critical for decision makings in both airline operations and air traffic management.
General Aviation Safety Analysis (FAA)
The objective of this FAA PEGASAS project was to analyze aircraft accidents and incidents that occurred at or near airports and to identify actual or potential airport risks and hotspots related to those accidents and incidents. Accidents and incidents were grouped into categories based on contributing factors and broken down by airport type. The results will provide insight into eliminating or mitigating risk factors that result in incidents and accidents.
Research Support
I gratefully acknowledge ongoing and past support from NSF, NASA, Airbus A^3, Rockwell Collins, Federal Aviation Administration, Virginia CCI, NVIDIA and Amazon AWS for my research group.
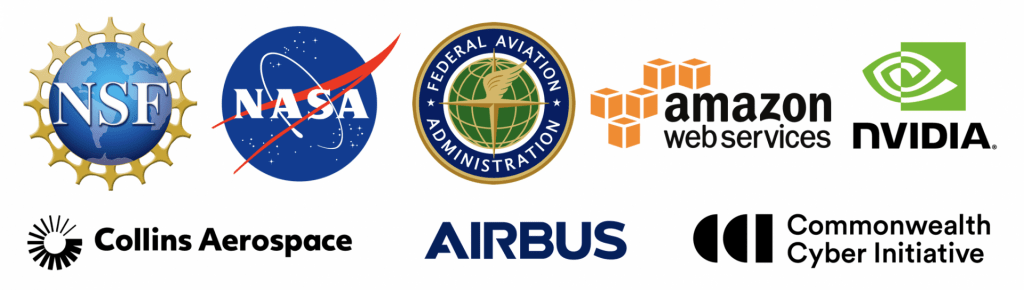